AI Accelerator Module Substrate Manufacturer.AI Accelerator Module Substrate Manufacturer refers to a company specializing in designing and producing substrates optimized for AI accelerator modules. They focus on enhancing computational efficiency, power management, and connectivity for AI-driven applications, ensuring high performance and reliability in advanced computing systems.
In our digital world, printed circuit boards (PCBs) play a vital role in the operation of countless electronic devices. With the rapid development of AI technology, AI accelerator module substrates have become a new focus. Understanding what AI accelerator module substrates are, their design and manufacturing processes, their application areas, and their advantages is crucial for anyone working in electronics or technology. This article aims to provide a comprehensive overview, covering everything from basic definitions to advanced applications.
What is AI Accelerator Module Substrate?
The AI accelerator module substrate is a specially designed printed circuit board designed to support and accelerate the calculation of artificial intelligence (AI) algorithms. As artificial intelligence and machine learning become more and more widely used in various fields, computing requirements are becoming more complex and larger. Traditional central processing units (CPUs) are often inefficient when processing these complex AI tasks and cannot meet the real-time and high-performance requirements. Therefore, the AI accelerator module substrate emerged as the times require and has become a key technology to solve this problem.
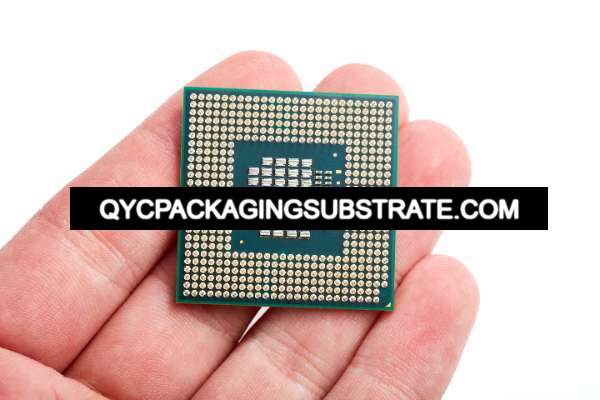
AI Accelerator Module Substrate Manufacturer
These substrates usually integrate a large number of processor cores, dedicated computing units (such as graphics processors (GPUs), tensor processing units (TPUs), etc.) and high-speed memories to optimize and improve the execution efficiency of AI tasks. The original design of the AI accelerator module substrate is to accelerate specific AI computing tasks, such as neural network training and inference, through hardware, thereby significantly reducing computing time and energy consumption.
First, the processor cores and dedicated computing units on the AI accelerator module substrate are capable of processing large amounts of data in parallel. Unlike traditional CPUs, these computing units have a highly parallel architecture and are capable of executing thousands or even millions of computing tasks simultaneously. This parallel computing capability is critical for deep learning and other complex AI algorithms, which often require processing a large number of matrix operations and data processing tasks.
Secondly, high-speed memory is another important component of the AI accelerator module substrate. These memories are used to store and quickly access large amounts of data and intermediate calculation results to ensure that processor cores and dedicated computing units can perform tasks efficiently. The existence of high-speed memory greatly reduces data transmission bottlenecks and improves overall system performance.
In addition, AI accelerator module substrates are usually equipped with advanced power management and heat dissipation designs to support the stable operation of high-performance computing units under high loads. Since AI computing tasks often generate large amounts of heat, advanced cooling solutions are key to ensuring system reliability.
AI accelerator module substrates are widely used in multiple fields, including data centers, autonomous driving, medical equipment, industrial automation and consumer electronics. For example, in data centers, AI accelerator module substrates are used to accelerate large-scale data processing and training of complex AI models; in the field of autonomous driving, these substrates help process vehicle sensor data and execute driving decisions in real time; in medical equipment, they are used Image recognition and diagnostic analysis.
In general, the AI accelerator module substrate significantly improves the execution efficiency of AI tasks by integrating high-performance processor cores, dedicated computing units and high-speed memory, and provides powerful hardware support for the development and application of artificial intelligence technology.
AI Accelerator Module Substrate design Reference Guide.
The AI Accelerator Module Baseboard Design Reference Guide is a comprehensive manual written for engineers and designers to guide them on how to create efficient and reliable AI accelerator module substrate layouts. This guide covers key steps from early design to final manufacturing, ensuring a smooth design process and high performance products.
First, the guide introduces best practices for AI accelerator module substrate design. These practices include component placement, signal integrity management, and thermal management strategies. Reasonable layout of components is crucial to minimize signal path length, reduce parasitic effects, and improve signal transmission efficiency. Signal integrity management involves the use of differential signal pairs, high-frequency design techniques, and appropriate shielding and grounding methods to reduce electromagnetic interference (EMI) and signal reflections. Thermal management strategies cover the use of heat sinks, thermal pads and thermal vias to effectively dissipate heat and ensure stable operation of high-performance components.
Next, the guide explains in detail the specific rules for AI accelerator module substrate design. These rules include electrical design rules and mechanical design rules. Electrical design rules involve trace width, spacing, impedance control, and power distribution network (PDN) design to ensure the electrical performance and reliability of the circuit. Mechanical design rules cover aspects such as substrate size, aperture, tolerance and stack-up structure to ensure the feasibility and consistency of the substrate during manufacturing and assembly.
The guide also introduces a variety of design software tools that are an integral part of modern PCB design. Commonly used software tools include Cadence Allegro, Altium Designer, Mentor Graphics Xpedition, etc. Each software has its own unique features and benefits, and engineers can choose the right tool based on project needs. These tools not only aid in circuit design and layout, but also provide simulation capabilities to verify the electrical and thermal performance of the design.
In addition, the guide highlights several key technologies to help engineers optimize the design of AI accelerator module substrates. These technologies include high-density interconnect (HDI) technology, micro-blind and buried via technologies, and flexible and rigid-flex board technologies. HDI technology makes it possible to achieve higher wiring density in a limited space and is suitable for miniaturized AI accelerator module design. Micro-blind via and buried via technology help reduce signal transmission paths and improve signal integrity. Flexible and rigid-flex board technologies provide solutions for applications that require bending and folding, such as wearable devices and space-constrained applications.
In summary, the AI accelerator module substrate design reference guide provides comprehensive guidance for designing efficient and reliable AI accelerator module substrates. By following these best practices, design rules, and using appropriate software tools and techniques, engineers and designers can ensure that the AI accelerator module substrates they design meet the highest standards in terms of performance, reliability, and manufacturing feasibility. This not only helps improve the computing efficiency and stability of AI systems, but also promotes the widespread application and development of AI technology in various fields.
What material is used in AI Accelerator Module Substrate?
AI accelerator module substrates often feature high-performance materials in their design and manufacturing, which are selected to meet their specific application needs. The main materials include high-frequency FR4, polyimide (PI) and fluorinated resin (PTFE).
High-frequency FR4 is a glass fiber-reinforced epoxy resin composite material with excellent electrical insulation properties and mechanical strength. It is suitable for applications requiring high-frequency and high-speed signal transmission. This material can not only effectively reduce signal loss, but also provide stable signal transmission characteristics, which is particularly important for AI accelerator module substrates.
Polyimide (PI) is a high-performance polymer with excellent high temperature resistance and chemical stability. It is often used in environments that require high temperature resistance and chemical corrosion resistance. In the AI accelerator module, PI materials can effectively manage heat, maintain stable operation of the circuit board, and have good mechanical strength, making it suitable for complex industrial and data center applications.
Fluorinated resins (PTFE) are known for their excellent electrical properties and chemical stability, maintaining stable performance over a wide temperature range. In the design of the AI accelerator module substrate, PTFE material can not only provide good signal integrity, but also effectively resist electromagnetic interference, ensuring the quality and stability of high-frequency and high-speed signal transmission.
The selection of these high-performance materials depends not only on their electrical and mechanical properties, but also on specific application requirements such as signal integrity, thermal management and environmental suitability. By rationally selecting and optimizing substrate materials, the performance and reliability of AI accelerator modules can be effectively improved, and the application and development of artificial intelligence technology in various fields can be promoted.
What size are AI Accelerator Module Substrate?
AI accelerator module substrates come in various sizes, depending on their application scenarios and design requirements. Different application scenarios have different requirements for the size and shape of the substrate. This article will explore these differences and the reasons behind them in detail.
In embedded systems and edge computing devices, AI accelerator module substrates are usually designed to be relatively small and compact. These devices require substrates that can be embedded into limited spaces while providing sufficient computing power to handle AI tasks. For example, smart cameras, drones, smart home devices, etc. all require high-performance but smaller AI accelerator modules. The size of such substrates may range from a few square centimeters to tens of square centimeters. This small size design not only contributes to the miniaturization of the equipment, but also reduces energy consumption and costs.
In consumer electronics such as smartphones and tablets, the size of the AI accelerator module substrate is also an important consideration. In order to ensure the portability and lightweight of the device, the substrate needs to be as compact as possible while maintaining high performance. Generally, the AI accelerator module substrate size in this type of equipment is also relatively small, but the design is more precise to meet higher performance and integration requirements.
AI accelerator module substrates in data centers and servers tend to be much larger compared to smaller devices. These systems need to process large amounts of data and complex AI computing tasks, so they require more powerful computing power and larger cooling space. This type of baseboard is usually designed as a large board that can be inserted into the expansion slot of the server and may be tens of centimeters or larger in size. For example, the substrate size of GPU accelerator cards used for deep learning training is usually larger to accommodate more processing cores and higher-capacity memory. These large substrates help improve computing efficiency and processing capabilities to meet the needs of high-performance computing in data centers.
In addition to the common applications mentioned above, some special application scenarios also have specific requirements for the size of the AI accelerator module substrate. For example, in medical devices, substrates need to conform to specific shape and size requirements to facilitate integration into the medical device. In the field of autonomous driving, the AI accelerator module substrate needs to adapt to the limited space inside the vehicle while having high shock resistance and high temperature resistance.
In general, the size of AI accelerator module substrates varies greatly depending on application scenarios and design requirements. Whether it is a small embedded system, a large data center, or various special applications, the size design of the substrate requires trade-offs in multiple aspects such as performance, cost, energy consumption, and heat dissipation. As technology continues to advance, the size and performance of AI accelerator module substrates will continue to be optimized to meet the growing demands for artificial intelligence computing.
The Manufacturer Process of AI Accelerator Module Substrate.
Manufacturing AI accelerator module substrates is a complex and precise process that requires precise control of multiple steps. The following are the main steps of the manufacturing process:
The substrate is usually made of high-performance materials such as FR4, polyimide (PI) or fluorinated resin (PTFE). These materials were selected for their excellent electrical properties and mechanical strength. First, the substrate is cut to the required size, cleaned and surface treated to ensure smooth subsequent processes.
A thin copper film is deposited on the surface of a substrate, usually by electroplating or chemical deposition. This layer of copper film will form the conductive path of the circuit board and is the basis for circuit connections.
Photolithography is the process of transferring circuit patterns to a substrate. First, a layer of light-sensitive material (photoresist) is applied to the copper layer. The circuit pattern is then exposed through a mask onto the photosensitive material. The exposed photosensitive material is removed during the development process, exposing the portion of the copper layer that needs to be etched.
Etching removes the copper layer not covered by photoresist. Commonly used etching methods include wet etching and dry etching. After etching, the remaining copper layer forms the desired circuit pattern.
According to the design requirements, drill holes in the substrate. These holes are used for through-hole mounting components (THT) and electrical connections. The drilling process usually uses laser drilling or mechanical drilling.
After completing the circuit pattern and drilling, start placing the components. Depending on design needs, choose surface mount technology (SMT) or through-hole mounting technology (THT). The SMT process is suitable for small and complex components, while the THT process is suitable for larger components that require stronger connections.
After the components are placed, they need to be fixed to the substrate by soldering. Commonly used soldering methods include reflow soldering and wave soldering. Reflow soldering is mainly used for SMT components. It uses heat to melt the solder and fix the components. Wave soldering is mainly used for THT components, and the component pins are welded to the substrate through molten solder waves.
Finally, the manufactured AI accelerator module substrate is tested. The test content includes electrical performance testing, functional testing and reliability testing to ensure that the substrate meets the design requirements and can work stably.
The entire manufacturing process requires a high degree of precision control and quality assurance to ensure the high performance and reliability of each AI accelerator module substrate. Through these meticulous manufacturing steps, the AI accelerator module substrate is able to provide excellent performance and stability in a variety of complex AI applications.
The Application area of AI Accelerator Module Substrate.
AI accelerator module substrates play a vital role in many frontier fields of modern technology. It is widely used in various industries, significantly improving the efficiency of data processing and artificial intelligence algorithm execution. The following are the main applications of AI accelerator module substrates in different fields:
Data centers are one of the most important application areas for AI accelerator module substrates. Data centers need to process massive amounts of data and execute complex AI algorithms such as deep learning and neural network model training. By integrating a large number of processor cores and dedicated computing units, these substrates can significantly accelerate data processing, improve computing performance, reduce latency, and optimize energy consumption to meet the efficient and reliable operation needs of data centers.
In the field of autonomous driving, AI accelerator module substrates are used to support real-time data processing and decision-making. Self-driving cars need to process large amounts of data from cameras, radars and other sensors for object recognition, path planning and dynamic decision-making. The AI accelerator module substrate ensures that the autonomous driving system can respond to complex road conditions in real time, improving driving safety and reliability by providing powerful computing power and low-latency data processing capabilities.
Image recognition and data analysis tasks in medical devices require very high computing power and accuracy. The AI accelerator module substrate can accelerate tasks such as medical image analysis, pathology detection, and genetic data processing, and improve diagnosis speed and accuracy. For example, in cancer screening, systems based on AI accelerator module substrates can quickly process and analyze large amounts of medical images, helping doctors make more accurate diagnosis and treatment decisions.
In the field of industrial automation, AI accelerator module substrates are used to improve the execution efficiency of machine learning models. Modern industrial automation systems rely on vast amounts of sensor data and complex algorithms to optimize production processes, monitor equipment health and perform predictive maintenance. The AI accelerator module substrate enables these systems to respond to changes faster and improve production efficiency and equipment utilization by accelerating data processing and analysis.
In the field of consumer electronics, AI accelerator module substrates are widely used in products such as smartphones, smart home devices, and wearable devices. AI functions in these devices, such as speech recognition, image processing and personalized recommendation systems, rely on efficient computing power. The AI accelerator module substrate can provide the required computing performance to ensure that these intelligent functions can quickly respond to user needs and provide a better user experience.
In short, the application of AI accelerator module substrates in data centers, autonomous driving, medical equipment, industrial automation and consumer electronics has significantly improved the application efficiency and effect of artificial intelligence technology, and promoted technological progress and innovative development in various industries.
What are the advantages of AI Accelerator Module Substrate?
AI accelerator module substrates have demonstrated a number of unique advantages in modern technology applications. Compared with other methods, they have significantly improved performance, customization, energy efficiency, reliability and development cycle.
First of all, high performance is one of the most significant advantages of AI accelerator module substrates. The AI accelerator module substrate is optimized for AI tasks and integrates a large number of processor cores and dedicated computing units. These hardware accelerators can significantly improve computing efficiency, allowing complex AI algorithms to be executed quickly. In fields such as data centers, autonomous driving, and medical image processing that require large-scale data processing and real-time response, the high performance of AI accelerator module substrates is particularly important.
Secondly, customization capabilities enable the AI accelerator module substrate to meet the needs of specific AI applications. Designers can specifically design and optimize modules based on specific AI application scenarios. This customized design not only improves the overall efficiency of the system, but also ensures that each module performs optimally for its specific task. For example, in autonomous driving, customized AI accelerator modules can better process real-time data and decision-making tasks, thereby improving vehicle safety and response speed.
High energy efficiency is another key advantage. The AI accelerator module substrate can significantly reduce power consumption while maintaining high performance through hardware acceleration. This is especially important for AI applications deployed at scale, such as data centers and IoT devices. The improvement in energy efficiency ratio not only helps reduce energy consumption and operating costs, but also conforms to the development trend of green environmental protection.
In terms of reliability, the AI accelerator module substrate uses high-quality materials and advanced manufacturing processes to ensure its long-term stable operation in various harsh environments. Whether in high temperature, low temperature or high humidity environments, high-quality materials and fine workmanship can ensure the stability and durability of the substrate. This reliability is especially important in medical equipment, industrial automation and other critical areas, ensuring that systems can continue to operate efficiently and reduce breakdowns and maintenance costs.
Finally, the modular design of the AI accelerator module substrate and mature design tools greatly shorten development and deployment time. Modular design makes the development process more flexible, and the design can be quickly adjusted and optimized to meet changing needs. Mature design tools provide complete design and simulation functions to help engineers quickly verify and optimize designs. This not only accelerates product time to market, but also improves development efficiency and product quality.
Overall, the AI accelerator module substrate has demonstrated significant advantages in terms of high performance, customization, energy efficiency, reliability and development cycle, making it a key component in promoting the application and development of AI technology. These advantages not only improve the overall performance and efficiency of the AI system, but also provide a solid foundation for technological innovation and application expansion in various industries.
FAQ
What is an AI accelerator module substrate?
The AI accelerator module substrate is a printed circuit board specially designed to optimize the computing efficiency of artificial intelligence algorithms. They usually integrate a large number of processor cores, dedicated computing units and high-speed memory to improve the speed and efficiency of AI task execution.
What materials are commonly used for AI accelerator module substrates?
Commonly used materials for AI accelerator module substrates include high-frequency FR4, polyimide (PI) and fluorinated resin (PTFE). These materials have excellent electrical properties, thermal stability and mechanical strength, making them suitable for high-frequency, high-speed signal transmission and strict thermal management needs.
How to determine the size of the AI accelerator module substrate?
The size of the AI accelerator module substrate depends on its application scenario and design requirements. For example, embedded systems and edge computing devices often require miniaturized modules, while data centers and servers may require larger boards to support more processing units and storage.
What are the main steps in manufacturing AI accelerator module substrates?
The manufacturing process includes substrate preparation, copper layer deposition, photolithography, etching, drilling, component placement, soldering and testing. High-end AI accelerator module substrates usually use surface mount technology (SMT) and through-hole mounting technology (THT) to ensure high-density wiring and high reliability.